As we ramp up to the release of Astera DW Builder, we’re looking to give readers an insight into how they can make their BI architectures faster, more agile, and ultimately better suited to the demands of today’s high volume, high-velocity data environment.
When it comes to building and implementing effective data warehousing solutions, few people can boast the kind of credentials that Paul Kellett brings to the table. With 25 years of experience under his belt working on a wide array of projects for organizations at every level, Paul has seen firsthand how clients have responded to the ever-growing need for business intelligence across their enterprise.
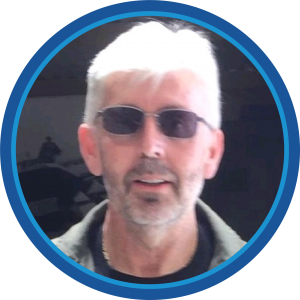
Paul Kellett (Data Warehousing Expert)
In this wide-ranging conversation, we took the opportunity to get some of Paul’s candid thoughts on such topics as – the current state of enterprise BI, how automation can accelerate data warehouse development, and where he sees the industry headed over the next couple of years.
So, what would you say are the most significant developments you’ve seen in enterprise BI since you began in this space?
So, I started in this industry 25+ ago with what was then called MIS (Management Information Systems), working predominantly in database projects. Since then, I’ve been involved in various BI projects from enterprise to departmental and SME.
If I were to talk about the major changes I’ve seen over that time, I’d say much greater awareness [of the importance of BI], greater data volumes, greater complexity around data, and improved supporting technology. While underlying costs and complexity are decreasing, there’s still a way to go there. [I think the] visual side of BI has also improved significantly.
What are some of the more common data warehousing use cases you see?
The variety of use cases is surprisingly broad but most typical are Greenfield environments where an organization has decided to implement BI or replacement scenarios for existing solutions. Replacement is typically driven by a poor/failed existing solution (which are surprisingly commonplace) or replacement of core source data systems. There are also a variety of use cases where elements of a solution are replaced altogether e.g. replacement of hand-coded ETL with an ETL tool. The latter tends to be more incremental.
Do you think every organization could benefit from a data warehouse?
A true data warehouse is not for everyone but I’ve yet to see an organization that could not improve its use of data and information.
An EDW can be a significant investment. So, [successful implementation of] BI normally requires cultural changes in how the business uses data. One of the common paths to “true BI” goes through data marts, departmental solutions, and improved reporting, with each subsequent implementation improving the organization’s use of data. An EDW lies at the end of this road in many cases.
Ironically, larger enterprises frequently end up with data mausoleums that bottleneck data delivery. [These architectures] are often highjacked by specific business units and therefore poorly support the broader business unlike a true enterprise solution [such as a data warehouse]. The solution lies in building more data marts to support different business elements. These data should then integrated with the core data warehouse.
Are there specific factors you’d look for when recommending an EDW to a client?
I look for a business with an appetite to improve its data awareness as everything should follow from that. I recommend a staged approach where possible. The end game could well be a data warehouse, but it’s important that business benefits are delivered progressively.
What are some of the key challenges that you see come up during the data warehouse development process?
Probably, accessing and understanding of data…that part is always fun. Getting businesses committed to the approach can also be an uphill task. Another constraint I come across is the lack of access to BI professionals who actually understand both the technology and can talk to the business.
What would you say is the most important step to get right during data warehouse development? Extracting source data? Modelling data? Creating ETL processes?
The most important part is the result. The data needs to be in a form that supports BI. Primarily, that means that data doesn’t lose any information and that it is available in a manner that is relevant to the business.
There are lots of ways to get to this point but it’s the destination that is most important. Having said that, the destination in BI is often unclear from the start, so the ability to quickly adapt the end result based on learnings and business feedback is paramount. This implies an effective and quick data delivery mechanism as well as a capable presentation tool [at the frontend].
What do you think about architectures such as the data lake or data vault? Are alternatives to the data warehouse, complementary solutions, or just a lot of hype?
Generally, these are complementary and not core. Data lakes are typically much cheaper and more straightforward of the two to implement. The concept of data lakes is relatively well established and has really just been re-branded in recent times. It might have been called an Operational Data Store (ODS) 20+ years ago. I frequently create an invisible data lake as part of a data warehouse solution. Invisible in that I don’t publish its existence as a data lake as there are flexibility and auditability benefits. A data lake can also be a cheap intermediate stage for a more fulsome BI solution e.g. it enables improved reporting.
A data vault is much more of an IT-driven component. More formal and structured, and IMO is less frequently justifiable because of the additional costs and timescales it adds to a solution. If there is a need to widely share common data across many applications in addition to the DW, then it becomes justifiable.
In your opinion, what role do data warehouse automation tools have in accelerating and streamlining data warehouse development?
Huge. Immense. Massive. Should I go on? Too frequently, a data warehouse is a monolithic, waterfall-type project in which us techno nerds are locked in a room for an extended period and asked to emerge with a best-guess solution of what we think the business needs. Yes, I exaggerate, but the process needs to be rapid and incremental with frequent short review\revise cycles that engage with the business. In other words, data warehousing needs to become truly agile.
From past experience, we know that breaking a DW down into many bite-sized chunks reduces risk, improves the quality of delivery, and improves engagement with the business. This is so much simpler to do with proper automation tools.
Another way to look at this is in terms of ROI. In the typical DW, the data preparation side makes up the bulk of the costs. Bringing these expenditures down with proper data-oriented automation has many benefits, from speedier delivery of better quality data to lowering the barrier to entry.
Improved quality is obviously a crucial benefit of automation as the amount that is bespoke, more complex, and prone to issues is reduced dramatically with an appropriate tool.
What features/functionalities do you think are essential for an effective data warehouse automation tool?
The repeatable tasks e.g., error handling, auditing, creation of SCDs, etc. need to be very simple and robust. There are a lot of repetitive tasks in a DW build. Integration into source systems and the destination model needs to be strong. For maintenance and agile delivery, the ability to do dependency analyses to understand processing is very important.
Do you think cloud data warehouses will replace on-premise EDW’s? Or do you see the data warehouse hybrid model being more prevalent?
Not sure. I see a general move to off-premise DWs as they provide greater flexibility, but that [cloud deployment] is not without its challenges. Probably all three will be common with a greater proportion of cloud DWs than on-premise.
Finally, where do you see the industry moving in the next five years or so?
The ‘sexy’ elements of BI, such as AI-driven analyses and more powerful visualizations, are receiving the bulk of focus. These elements will probably continue to dominate the space. However, I see a quiet revolution on the data delivery side. With the availability of data taking more and more importance as organizations realize it is usually the largest cost.
Take a First-Hand Look at the Future of Data Warehousing
The time to modernize your enterprise BI is now. With Astera DW Builder, you can leverage a complete end-to-end platform that promises to make the design, development, and deployment of your EDW lightning fast. Find out how we can support your use case, contact our technical experts today.