Unlock the full potential of your supply chain data with AI-powered document data extraction and unleash unprecedented efficiency, accuracy, and competitive advantage!
Navigating the complex world of supply chain data can be overwhelming, especially when grappling with vast amounts of unstructured and semi-structured information. Conventional data extraction methods often struggle to keep up with the ever-growing volume of data.
Enter artificial intelligence (AI), a game-changer that’s set to revolutionize the way organizations handle document data extraction in supply chain management. With AI, professionals in the field can now unlock the full potential of their supply chain data, making intricate extractions a breeze. The impact of AI in supply chain management is backed by impressive numbers. A Gartner study predicts that Through 2024, 50% of supply chain organizations will invest in applications that support artificial intelligence and advanced analytics capabilities.
Let’s take a dive into supply chain document extraction, understand its challenges, and discuss the best ways to ensure seamless document processing.
Decoding Diverse Supply Chain Documents
The supply chain ecosystem has a wide variety of documents, each packed with critical data that plays an essential role in managing and optimizing business operations. Let’s take a closer look at some of the most common types of documents in the supply chain industry and their significance.
Invoices and Purchase Orders
Invoices and purchase orders (POs) are the lifeblood of the supply chain, as they facilitate the exchange of goods and services between suppliers and customers. The Invoices detail the products or services provided, their quantities, and prices, while purchase orders are official requests made by buyers to procure specific goods or services. Extracting accurate data from these documents is crucial for ensuring smooth transactions, managing vendor relationships, and maintaining accurate financial records.
Shipping and Receiving Documents
Shipping and receiving documents, such as bills of lading, packing slips, and delivery notes, serve as essential records for the transportation and logistics aspect of supply chain management. These documents contain vital information about shipment details, including the carrier, the sender and receiver, item descriptions, and quantities. Efficiently extracting and managing this data is key to ensuring that goods are transported and delivered accurately, on time, and in compliance with various regulations.
Inventory Reports and Forecasts
Inventory management is a critical component of supply chain operations, and it relies heavily on accurate and timely data from inventory reports and forecasts. These documents provide crucial insights into the status of stock levels, product demand, and inventory turnover. By extracting data from inventory reports and forecasts, businesses can gain valuable insights to make informed decisions about reordering, stock replenishment, and demand planning. This helps to reduce the risk of stockouts or overstocking, ultimately contributing to better supply chain efficiency and profitability.
Navigating Data Extraction Roadblocks in Supply Chain Data Management
As the supply chain industry thrives on a wealth of data, organizations must overcome several challenges when extracting valuable information from documents. Let’s explore some of the most prominent obstacles in document data extraction for supply chain functions and how they impact operations.
Handling Unstructured and Semi-Structured Supply Chain Data
A significant portion of supply chain documents, such as invoices, purchase orders, and shipping documents, come in unstructured or semi-structured formats. This makes it difficult to extract data using traditional, rule-based extraction methods, as they require consistent patterns and structures to function effectively. Consequently, managing unstructured and semi-structured data becomes a major challenge, as it demands additional time, resources, and expertise to ensure accurate and efficient extraction.
Time-Consuming Manual Processes
Manual data extraction, although a prevalent method, can be incredibly time-consuming and labor-intensive. Supply chain professionals often find themselves sifting through stacks of documents, manually entering data into various systems or spreadsheets. This tedious process not only eats up valuable time and resources but also diverts focus away from more strategic and value-added tasks. As a result, organizations risk losing their competitive edge in an ever-evolving market landscape.
Inaccurate and Error-Prone Results
Manual data extraction is inherently susceptible to human error, leading to inaccuracies and inconsistencies in the extracted data. These errors can have far-reaching consequences, impacting everything from financial records to inventory management and logistics planning. Moreover, inaccuracies in data can cause discrepancies between different systems, making it difficult to maintain a single source of truth across the organization. This lack of reliable data hinders informed decision-making, ultimately impairing the efficiency and effectiveness of supply chain operations.
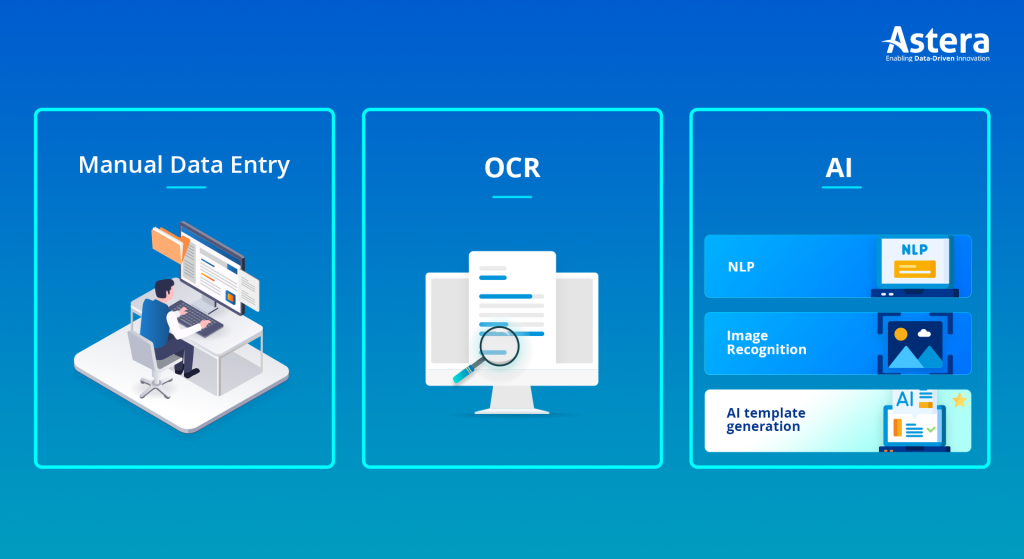
Supply Chain Data Extraction Techniques: A Glimpse
Supply chain professionals have a myriad of document data extraction techniques at their disposal. Each method comes with its own set of benefits and drawbacks. Let’s dive into the most common approaches and how they stack up against each other.
Manual Data Entry
The traditional approach to document data extraction, manual data entry, involves individuals meticulously scanning through documents and manually inputting the necessary information into a designated system or spreadsheet. While this method may be suitable for small-scale operations, it’s labor-intensive, time-consuming, and prone to human error, making it less than ideal for larger organizations dealing with high volumes of data.
Optical Character Recognition (OCR)
Optical Character Recognition (OCR) technology takes data extraction a step further by converting printed or handwritten text into machine-encoded text. This allows for the automated extraction of information from documents, significantly reducing the manual labor involved. Although OCR is a considerable improvement over manual data entry, it’s not without its limitations. The technology struggles with poor-quality images or inconsistent text formatting, and it’s also limited in handling unstructured or semi-structured data.
AI-Based Document Data Extraction
AI-based document data extraction is a cutting-edge approach that leverages the power of artificial intelligence and machine learning algorithms to extract information from a wide variety of document formats. This method brings a new level of efficiency and accuracy to the data extraction process, making it the go-to-choice for many organizations in the supply chain industry. Here are a few notable AI-based document data extraction techniques:
- Natural Language Processing (NLP): NLP allows computers to understand, interpret, and generate human language in a way that’s both meaningful and valuable. NLP can extract data from text-heavy documents effectively, but it might not be as efficient when dealing with structured data in tables or invoices.
- Image Recognition and Processing: AI-driven image recognition and processing techniques can identify and extract specific data elements from images or scanned documents. However, they can struggle with complex or inconsistent document layouts, which are common in supply chain documents.
- AI Template Generation: This advanced method of AI-based document data extraction involves the automatic creation of extraction templates using machine learning algorithms. AI template generation is designed to handle a wide range of document formats, including unstructured and semi-structured data. It significantly reduces the time and effort required to create extraction templates and allows for easy adaptation to different document layouts.
AI template generation emerges as the most effective AI-based document data extraction method, primarily because it combines the best aspects of NLP, image recognition, and processing techniques.
Embracing the Future of Supply Chain Data Management
In a rapidly evolving global market, embracing AI-powered document data extraction is more than just a competitive advantage; it’s a strategic necessity.
By integrating AI-driven techniques like AI template generation into supply chain operations, businesses can overcome the challenges associated with traditional data extraction methods and unlock the full potential of their supply chain data.
So, take the leap and revolutionize your supply chain data management practices with AI-powered document data extraction – the future is now!
Simplify Supply Chain Data Extraction with Astera ReportMiner
Astera ReportMiner is a powerful AI-based solution tailored for the supply chain industry that simplifies the complex task of extracting data from a wide range of supply chain documents. With its intuitive and no-code interface, businesses can easily automate their data extraction processes and eliminate the need for manual data entry.
From invoices and purchase orders to shipping documents and inventory reports, ReportMiner can accurately capture data from any unstructured or semi-structured document and convert it into a structured format. This results in reduced errors and increased accuracy, leading to better decision-making in supply chain management.
Astera ReportMiner streamlines document management processes in your supply chain, reduces time and costs, and maintains a competitive edge in the constantly evolving market landscape.
Try out ReportMiner or talk to our sales team about your requirements in supply chain data management.
Authors:
Ovais Naseem